article
Introspective Conversational AI: A self-learning AI chatbot that talks to itself
April 29, 2022 • 7 minutes
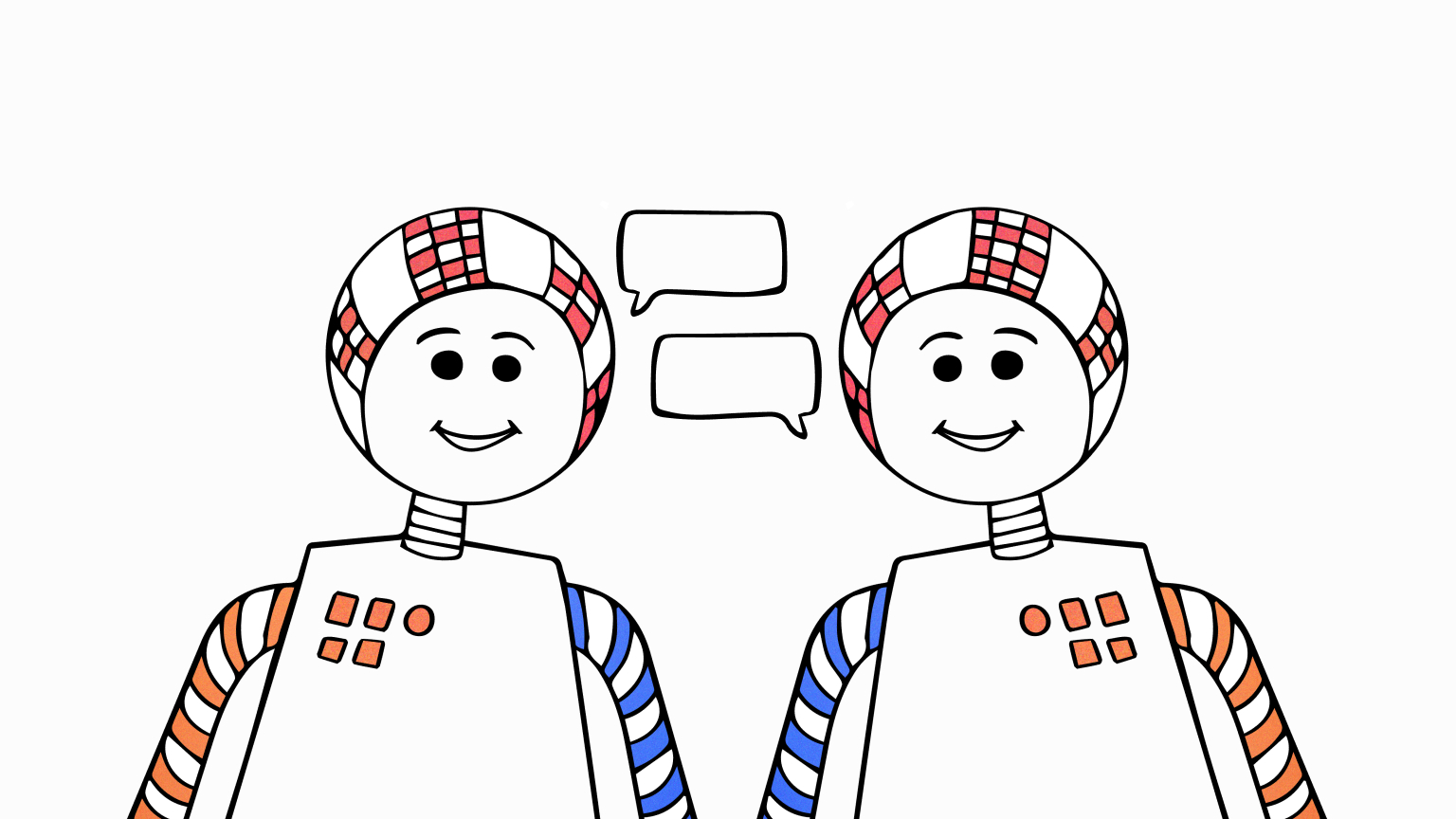
The scale of digital consumption increases every year, and the power to make or break a brand is in the hands of an estimated 2.1 billion consumers. To handle increased traffic, but still provide excellent conversational customer service, enterprising companies are increasingly relying on virtual agents. Also known as a virtual assistant or AI chatbot, they can be programmed to help with customer inquiries, from ordering a burrito to sending and receiving crypto. Unfortunately, these virtual agents frequently have conversations like the one below. Such conversations can lead to mismanaged conversations that create frustration, or potentially permanent damage to the brand and a ruined customer relationship.
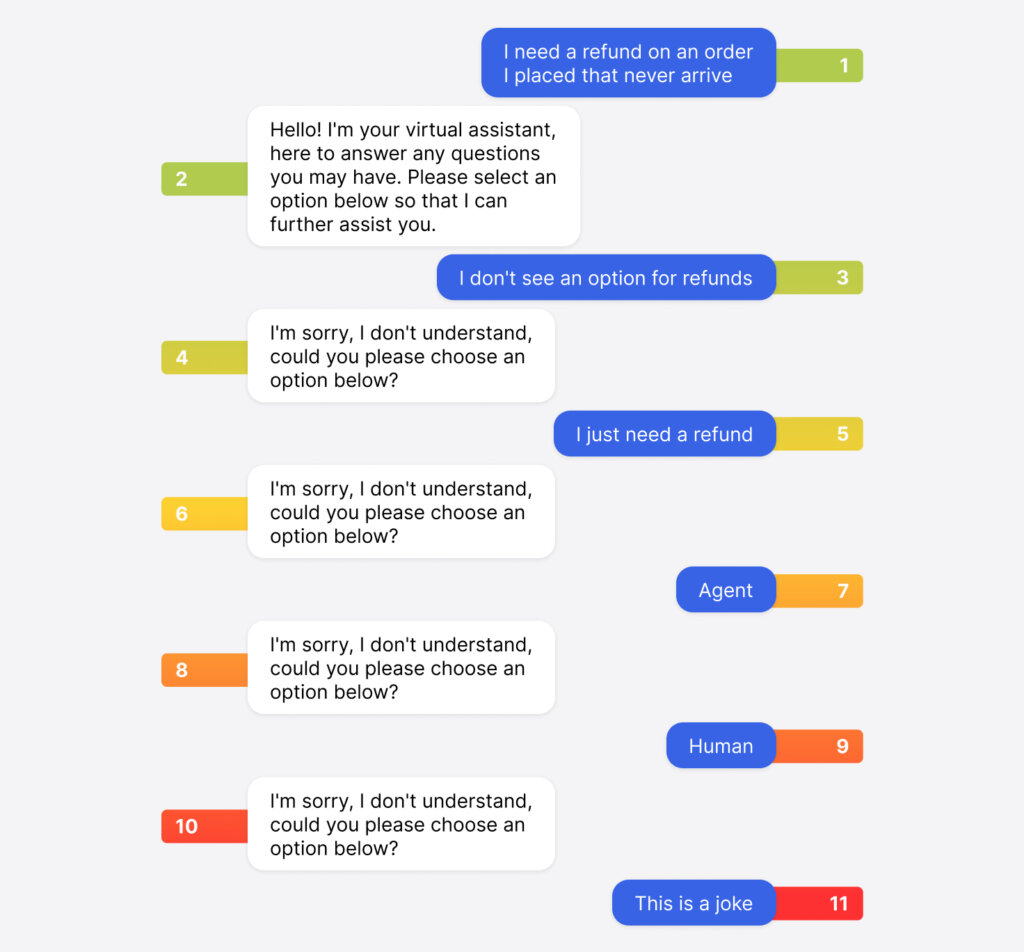
Most virtual agents rely on scripts alone
Psychology research tells us that people self-monitor (Snyder, 1974), or introspect on their social behaviors and change what they say accordingly. For example, someone may notice their supervisor prefers indirect questions to direct ones. In the workplace, self-monitors “get along” and “get ahead” — they’re better liked and promoted more often (e.g. Day & Schleicher, 2006).
Typical virtual agents cannot self-monitor by design like human agents; they are built to follow static, predefined scripts. In most conversations, the virtual agent limits the consumer to select from a few menu options. Any response the virtual agent can take, must be predicated on a discrete customer action or user intent. Even a virtual agent powered by the world’s most advanced NLP / NLU engine must have pre-built agent responses individually assigned to a customer’s intent. With each new path the customer can take within a conversation, the script complicates exponentially, increasing the time and effort to both build and maintain these convoluted scripts. As a consequence, many brands end up sacrificing quality for simplicity and scalability.
LivePerson’s intelligent virtual agents introspect: One step closer to a self-learning AI chatbot
The cost of a bad virtual agent experience is immediate and irreparable; 73% of consumers will ghost a brand after just three or fewer poor customer experiences. Because of the human tendency to anthropomorphize anything and everything, customers end up applying the same social rules and expectations to machines and artificial intelligence technology as they do to people (Nass et al., 1994). Self-monitoring virtual agents (what one might call a self-learning AI chatbot) are required to bridge the gap between existing machine-learning capabilities and our expectations for bots to behave like people. For example, a virtual agent could be more empathetic when interacting with frustrated consumers like in the example above.
Introspection is the ability to reflect on ones’ own behaviors, feelings, and thoughts. It is the crucial first step for active self-monitoring in a self-learning AI chatbot. Not only would introspection allow virtual agents to critique their own performance in individual conversations, it would empower them to identify larger patterns across their conversation history. For example, the agent might learn that consumers get angry about shipping delays due to poor weather, which is completely outside of its control. However, the agent may discover across many conversations that it can alleviate these customers’ frustrations by providing sincere apologies. The virtual agent could present these findings to the chatbot development team, who would then adjust the virtual agent’s dialogue for the appropriate context accordingly.
LivePerson recently released an AI function that enables virtual agent introspection. First, it identifies conversations that contain issue types, or frustrating events. For instance, in the example conversation, the virtual agent Doesn’t Understand the consumer. Once all the issue types are identified, the pipeline rates the customer’s experience during that conversation on a scale of 1-3. The score becomes MACS — the Meaningful Automated Conversation Score.
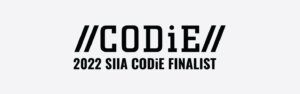
LivePerson’s Meaningful Automated Conversation Score (MACS) is a Finalist in the 2022 CODiE Awards for the “Best Business Intelligence Solution”
Minimum effort, MACS-imum impact
Before MACS, there were three ways to evaluate and improve virtual agents. Tuners — people who improve scripts — could read hundreds or thousands of conversations, searching for relevant information and potential insights. Alternatively, virtual agents could get user feedback by asking consumers to fill out a survey after their problem is resolved. However, less than half of angry customers voice their complaints directly to a brand. Lastly, tuners could use sentiment analysis algorithms. These algorithms are great at identifying if consumers express frustration, but they are unable to identify the root cause of the problem, let alone a possible solution. In contrast, MACS is the first low-effort, comprehensive, and actionable measure of consumer experience.
MACS streamlines how companies manage conversational chatbots. From the LivePerson Bot Analytics dashboard, tuners can use MACS to immediately target their poorest-performing bots for corrective actions.
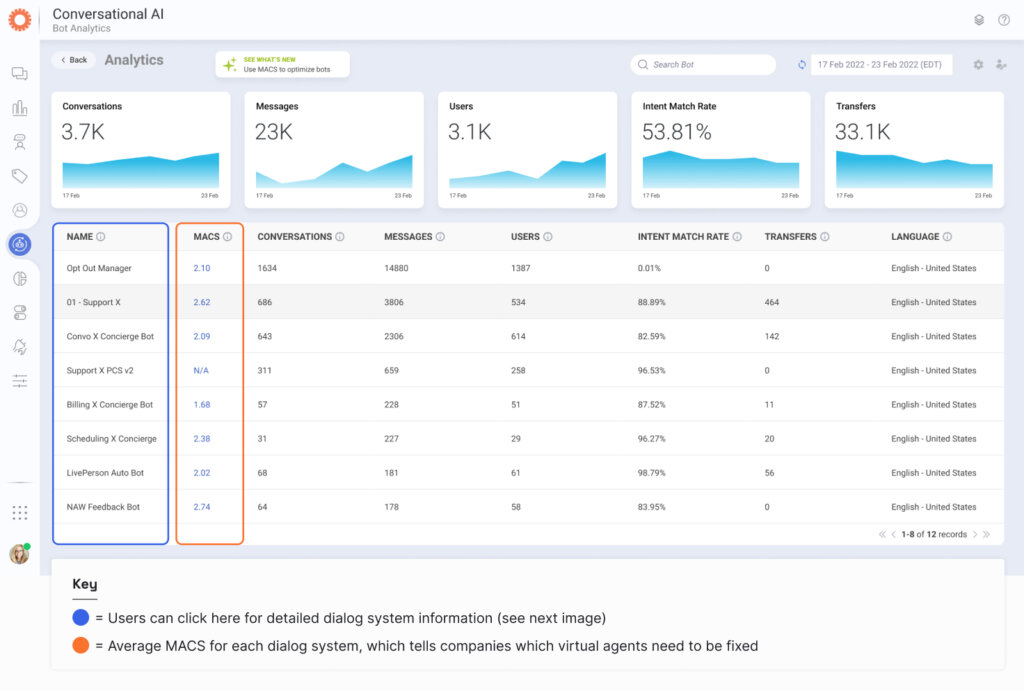
MACS also provides detailed information for each bot agent. The MACS detail page is designed to provide immediate answers to common questions:
- How is the bot performing (trend line)?
- When and where is the bot failing to provide a positive customer experience (intent and interaction graph)?
- And most importantly, why is the bot unable to provide a positive experience and how can it be resolved (issue types)?
The insight that used to take large amounts of time to find via reading sample conversations, now takes just a few clicks.
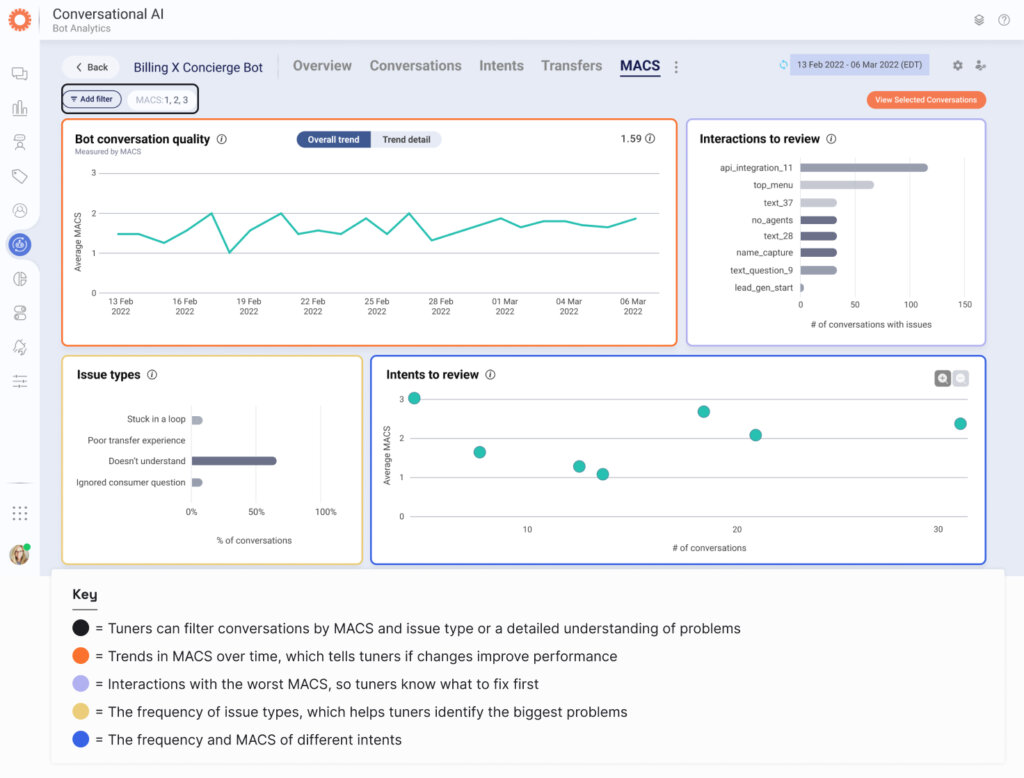
MACS in (M)ACS-tion
The virtual agent pictured above had a stable but undesirable MACS of approximately 1.6. At first glance, it is obvious the biggest problem was that it did not understand consumers, especially during the “api_integration_11” interaction. After a minute of looking at these graphs, a human agent or chatbot tuner already knows what action they can take to make the biggest positive impact on this virtual agent experience: improve the understanding of the agent in the API integration interaction.
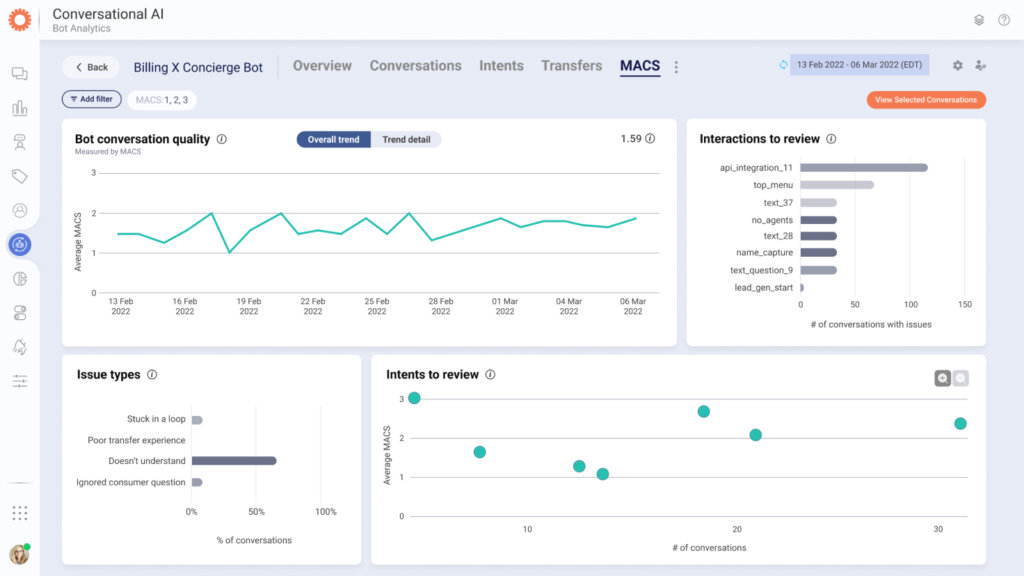
After improving the virtual agent’s understanding, the “doesn’t understand” issue type is less frequent, and the MACS score has increased to approximately 2. Consumers are having better conversations, which leads to more positive relationships with the brand. While “doesn’t understand” still occurs, MACS has helped the tuner improve the script substantially, in the time it would take to read a single conversation.
LivePerson is now one step closer to a self-monitoring, self-learning AI chatbot. Since these virtual agents can introspect, tuners will spend more time implementing impactful solutions and more complex tasks, instead of mining for potential insights. LivePerson will not stop here, and is already working on the next version of MACS. With this next version, planned for late 2022, virtual agents will begin to be able to tune their own scripts, either in pre-approved ways in the moment (e.g. empathizing or offering to transfer), or by asking tuners to approve bigger changes to the script. Virtual agents will even be able to evaluate their scripts before deployment, providing their own quality assurance. Bots will be better, tuners will be more efficient, and 2.1 billion people will be happier.
How can you learn more?
Request a consultation to see how automation, driven by MACS, can help your business succeed, or read up on technical details below: