article
How to discover the ROI of real-time agent assist AI tools
From customer service challenges to AI-powered agent productivity solutions using LivePerson’s Expected Net Cost Savings model
July 10, 2023 • 9 minutes
July 10, 2023 • 9 minutes
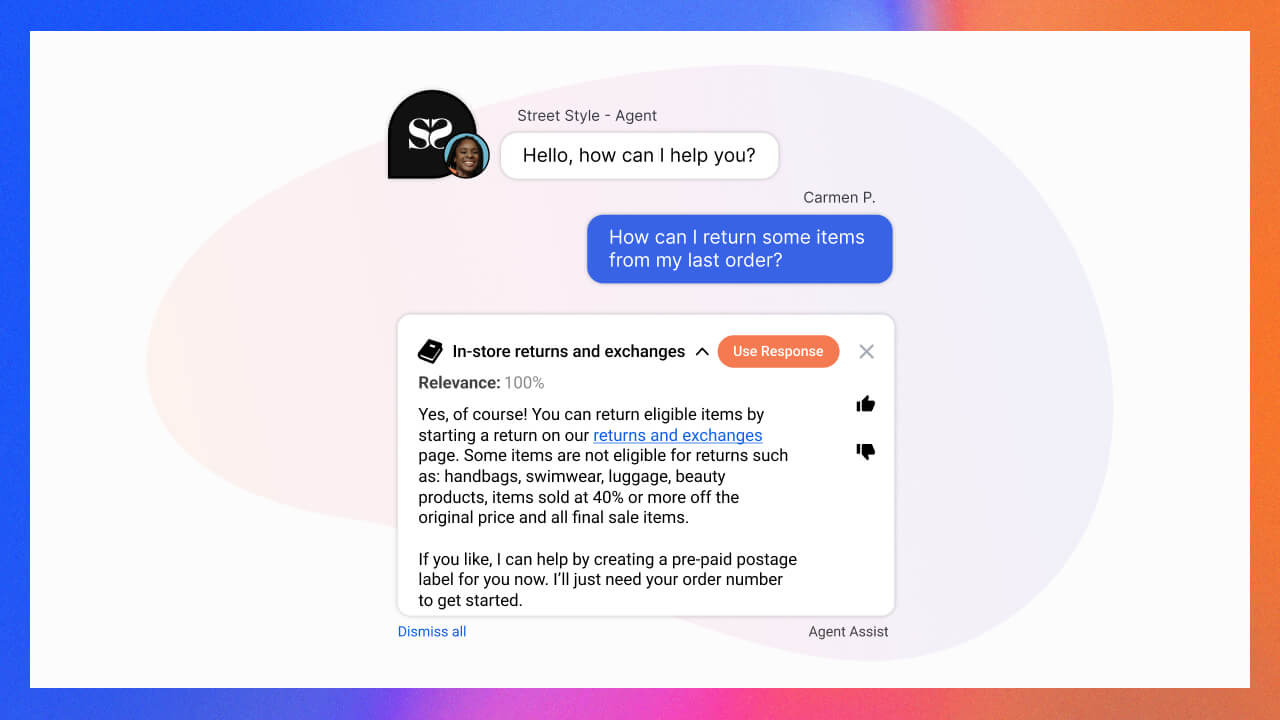
Over the past few months, generative AI has gone mainstream. Since the launch of ChatGPT in November 2022, hundreds of millions of people have interacted with large language models (LLMs), a type of generative AI trained on vast amounts of text, which can follow instructions and generate natural language with near human-like abilities. ChatGPT made LLMs widely accessible and easy to use, allowing individuals to spark conversations, ask questions, and craft content in practical ways that we’d never imagined. This pivotal technology has rapidly found its way into many applications that are beginning to change how businesses operate, transforming a wide range of verticals, from retail to healthcare, while touching nearly every department, from marketing to customer service.
Businesses are quickly realizing the transformative potential of LLMs, with conversational customer service being one of the first areas to benefit. Customer service remains one of the most resource-intensive departments of any business, posing significant operational challenges in terms of cost and time. Contact center agents often find themselves manually sifting through vast amounts of content to find the right answer to a customer’s issue. On top of that, they are expected to handle multiple conversations simultaneously while each customer anxiously waits and expects immediate responses. LLM-powered generative AI tools naturally address these challenges by equipping agents with instant, contextually relevant responses that streamline live customer interactions, leading to significant cost savings and enhanced efficiency. However, it’s essential to weigh their effectiveness against the costs of training and deployment.
In response to this, LivePerson introduced the Expected Net Cost Savings Model (ENCS) to provide a clear, practical view of the value and return on investment from deploying Conversation Assist, our LLM-powered agent assistance tool.
In this blog post, we’ll unpack how we can measure the impact of LLMs, like those from Cohere and OpenAI, with LivePerson’s Conversation Assist, using our Expected Net Cost Savings Model. We’ll look at three strategies for customizing these models based on our collaboration with a leading retailer. Plus, we’ll explore how ENCS isn’t just a static tool — it’s a dynamic framework designed to adapt to future models and products.
Boosting agent productivity with LLMs: LivePerson’s AI-powered Conversation Assist
During our generative AI launch in April, we unveiled Conversation Assist, a real-time agent assist tool designed to supercharge agent productivity. This tool, powered by LLMs, provides agents with contextually relevant answer recommendations, intelligently generating responses based on a brand’s specific data. Businesses can feed their knowledge base articles, internal documents, and website content into the LLM, which then uses this information to generate responses. The tool also factors in prompt configuration controls and the context from a conversation to produce a recommended result. Once a response is generated, human agents are in the loop with the ability to review, edit, and approve it before it reaches the customer, ensuring accuracy and relevance in every interaction.
This real-time agent assist has led to significant benefits for LivePerson customers, including:
- Enhanced agent productivity and lower cost per conversation: Our customers have seen a significant improvement in their agents’ ability to respond to routine questions and efficiently address customer needs, resulting in faster resolution times and significant cost reductions per conversation.
- Reduced agent burnout: With instant access to relevant and up-to-date responses, agents can shift their efforts from manual, time-consuming tasks. The LLMs handle the heavy lifting, allowing agents to focus on more complex, higher-value tasks.
- Higher CSAT: Customers have found that responses are more consistent and relevant across all customer interactions, resulting in higher customer satisfaction.
Measuring the impact: Meet the model that proves saving agents time can increase cost savings
In our ongoing mission to deliver solutions that drive better business outcomes and boost our customers’ bottom lines, LivePerson’s Data Science team developed the Expected Net Cost Savings Model (ENCS). This unique, straightforward, and adaptable framework measures the impact of Conversation Assist. ENCS is a framework that combines the likelihood and cost savings from an agent accepting or editing an LLM-powered recommendation with the cost of generating such a response. It takes into account the model’s performance, the cost of the model, and the labor cost of a human agent.
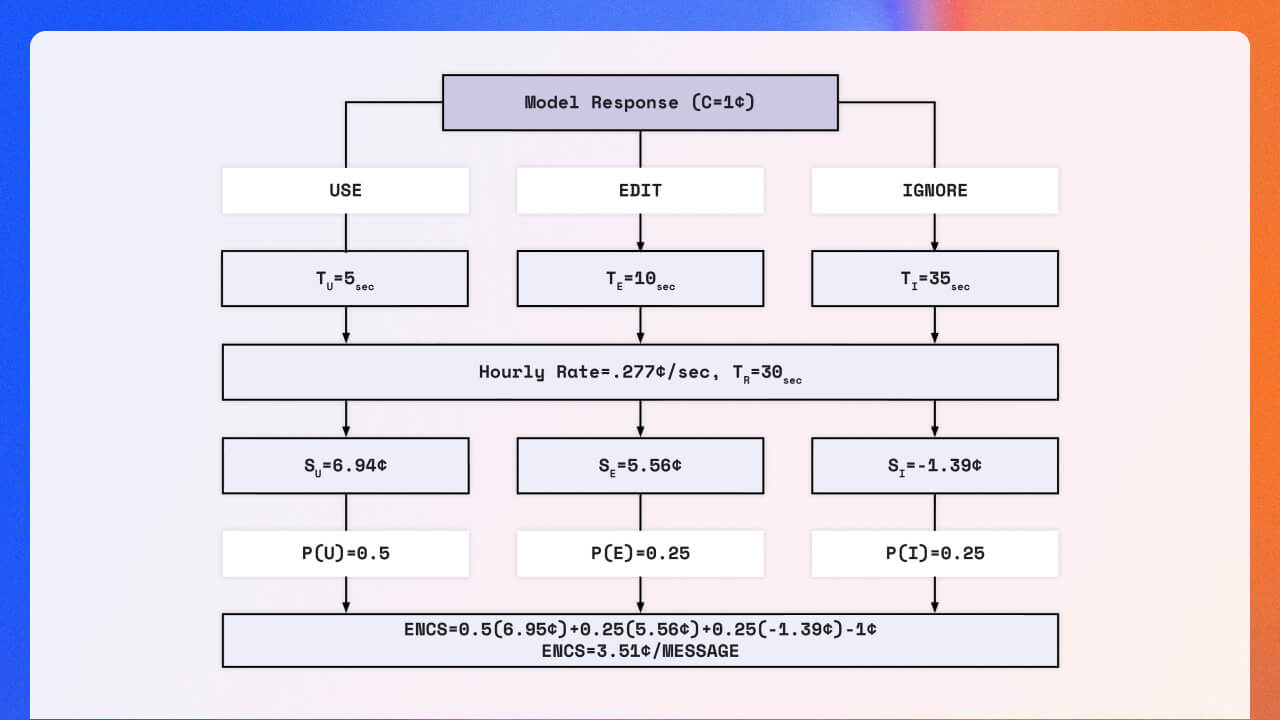
Think of it this way: each time an agent uses a response generated by Conversation Assist, the business stands to save money. ENCS calculates these potential savings by considering how likely the response will be used and subtracting the cost of generating the response. However, agents don’t always use responses in their original form. They may edit the suggested answers to better fit their context, or they might disregard them altogether. ENCS takes this into account, factoring in the likelihood of an agent editing or ignoring a response, along with the associated savings and costs. As a result, models optimized for accuracy versus efficiency can have different impacts on a business’s bottom line.
Footnote: It’s important to note that ENCS is based on the premise that agents are always engaged in active conversations or other essential tasks. It doesn’t account for broader complexities such as workforce optimization or inherent research and development costs. Additionally, it doesn’t consider potential costs that could arise from an agent using an inappropriate or factually incorrect response.
Putting theory into practice: An example of real-time agent assist value
In February 2023, we partnered with a leading retailer to pilot Conversation Assist and gain insight into which model could yield the most significant cost savings using ENCS. We equipped their agents with the tool and allowed them to rate the recommended responses. The retailer’s customer base includes both consumers and sellers who consign luxury items through their platform. This diverse customer base presents a wide variety of issues for customer service agents to address, providing us with a rich collection of feedback across a broad spectrum of data. On average, the retailer handles around 15,000 customer service conversations per month, with about 350 agents sending an average of 100,000 human-authored messages monthly through LivePerson’s Conversational Cloud.
LivePerson’s Data Science team experimented with three different strategies to customize the models: prompt engineering, fine-tuning, and knowledge distillation. These strategies were tested across eleven different configurations using Cohere’s XLarge and OpenAI’s GPT-2 and GPT-3 models. From these, they selected three setups and gathered feedback from the retailer’s agents on the model outputs. The team then worked with nine retail agents already familiar with our Conversation Assist tool and asked them about their likely actions concerning the recommended responses: Would they use them as is, modify them, or disregard them entirely?
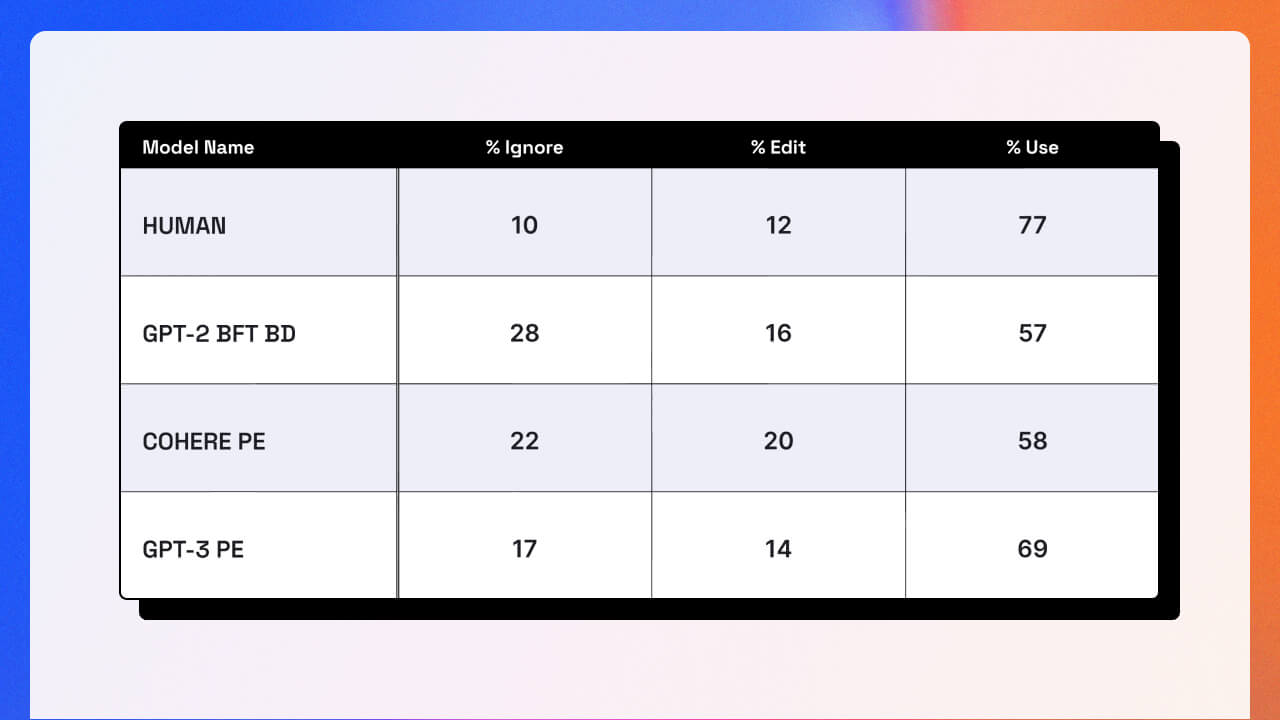
In addition to sharing recommended responses from models, our team also had the agents rate actual responses that another human agent had sent to a customer. Interestingly, even when presented with a reply used in a real conversation, agents chose to use the reply as it was only 77% of the time, highlighting the significant role personal preferences play in an agent’s choice of responses. This practical insight helps us gauge the highest usage rate we could expect from a model.
The team used an automated metric known as ‘perplexity’ to estimate usage rates for additional model configuration strategies. They applied this to every conversation scenario, the responses generated by each LLM, and the predicted response usability scores. These insights, coupled with the cost of generating a response for each LLM, were then fed into the ENCS model. This allowed them to calculate potential cost savings per message and per year across all 11 different model configurations. The results were promising: These approaches could lead to an agent usage rate of 83%, either directly or through editing, with annual cost savings of $60,000, which makes up 60% of their entire budget for staffing their agents.
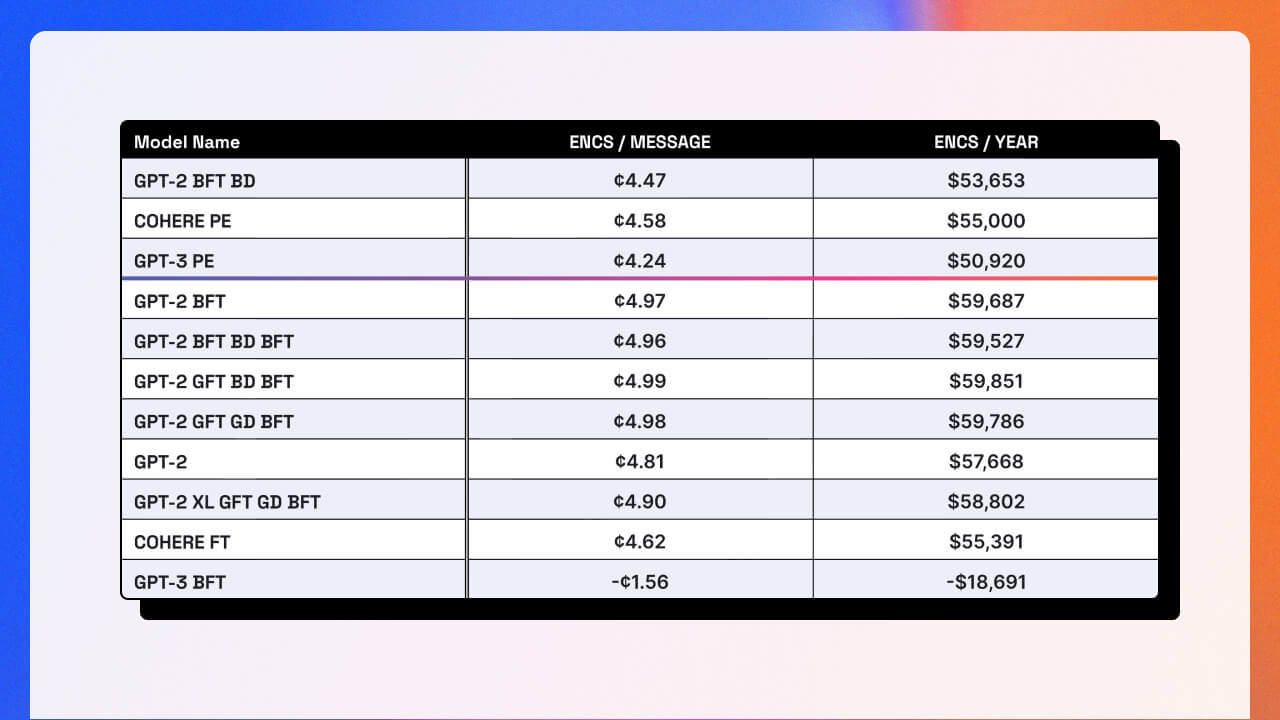
These results show that the annual cost savings between these models are not significantly different. The in-house GPT-2 model, while producing less useful outputs, is cheaper to serve and results in slightly more savings than the GPT-3 model. The GPT-3 model, though more frequently used by agents, is considerably more expensive. With ENCS, we can compare these factors side-by-side, balancing cost savings with other considerations like the value of owning and controlling a model versus relying on a third party. This becomes increasingly valuable as technology continues to evolve.
Navigating the rapidly evolving LLM landscape with ENCS
Since the inception of LivePerson’s ENCS in February 2023, the LLM landscape has been anything but static. LLM leaders like OpenAI and Cohere have been advancing quickly, unveiling more powerful models than the ones used in our ENCS testing. Meanwhile, a new wave of open-source LLMs has emerged, offering improved performance through fine-tuning, reducing computational overhead, and ultimately driving down costs. In this swiftly changing landscape, ENCS serves as a tool designed with model-agnostic principles at its core. ENCS empowers business leaders to invest in the most cost-effective models and products that deliver tangible benefits tailored to their unique needs, even in the face of evolving technology and fluctuating pricing structures.
After introducing LivePerson’s ENCS model, our Data Science team has been rigorously testing against new open-source models, including LLaMa, Vicuna, and Falcon. They’ve found that these models produce far better outputs than GPT-2, and while they are more expensive to serve, technological advancements are quickly reducing this cost. For ENCS, an in-house LLM can have a response usability rate much closer to that of third-party models like GPT-3 and Cohere, but at a similar or lower cost to serve. This rapid progress in the availability and usability of open-source LLMs over the past four months underscores the value of a flexible cost-savings model. As technology progresses and the capabilities and costs of LLMs evolve, a framework that balances these factors enables us to drive better business outcomes.
Conclusion: The strategic value of LLMs and ENCS
While LLM-powered tools are poised to drive better business outcomes for customer service, it’s crucial to quantify their value and return on investment to ensure these real-time agent assist technologies deliver measurable, meaningful results tailored to specific business needs and use cases. ENCS provides a timeless and universal framework, empowering businesses to make strategic investments in these ever-evolving technologies.
This blog was based on a paper entitled, “The economic trade-offs of large language models: A case study,” authored by Kristen Howell, Gwen Christian, Pavel Fomitchov, Gitit Kehat, Julianne Marzulla, Leanne Rolston, Jadin Tredup, Ilana Zimmerman, Ethan Selfridge, and Joseph Bradley.
Customer Exclusive Offer: Try out Conversation Assist for free today!
Log in to Conversational Cloud and sign up in the management portal.