article
Traditional KPIs do not work as chatbot metrics
How to shift the perspective of key bot metrics to better measure chatbot performance
August 25, 2022 • 6 minutes
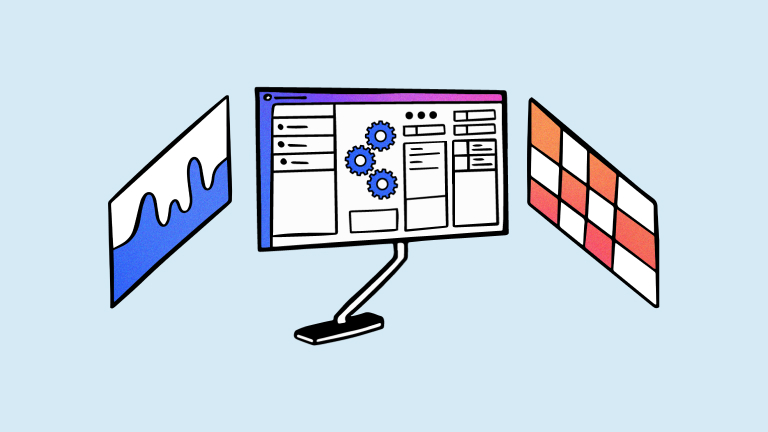
Automation and artificial intelligence provide amazing benefits when used in digital engagement programs. We continue to see expanded use of bots and automation in chat, messaging, voice, and even social conversational programs. By 2024, Insider Intelligence predicts that consumer retail spend via chatbots worldwide will reach $142 billion — up from just $2.8 billion in 2019.
At LivePerson we already see over 70% of all conversations on our Conversational Cloud® using some type of automation, a trend that shows no sign of slowing down. That means we need to assess chatbot success properly, but that doesn’t always mean leaning on familiar key performance indicators (KPIs).
When new human agents walk through the door, we don’t just immediately turn them loose on customers. They are trained and managed, and bots should be afforded the same courtesy, whether they are used for simple tasks — routing to a human agent or collecting info — or complex ones — selling a grill or handling insurance claims. Bots need to be monitored, nurtured, and trained over their lifetime, the same as humans.
This means measuring chatbot performance with metrics designed for them. Too often I hear leaders using KPIs like containment, customer satisfaction metrics (CSAT or NPS), sentiment, and other key metrics in their bot analytics dashboard.
The problem? These traditional key metrics just don’t work. Here’s why:
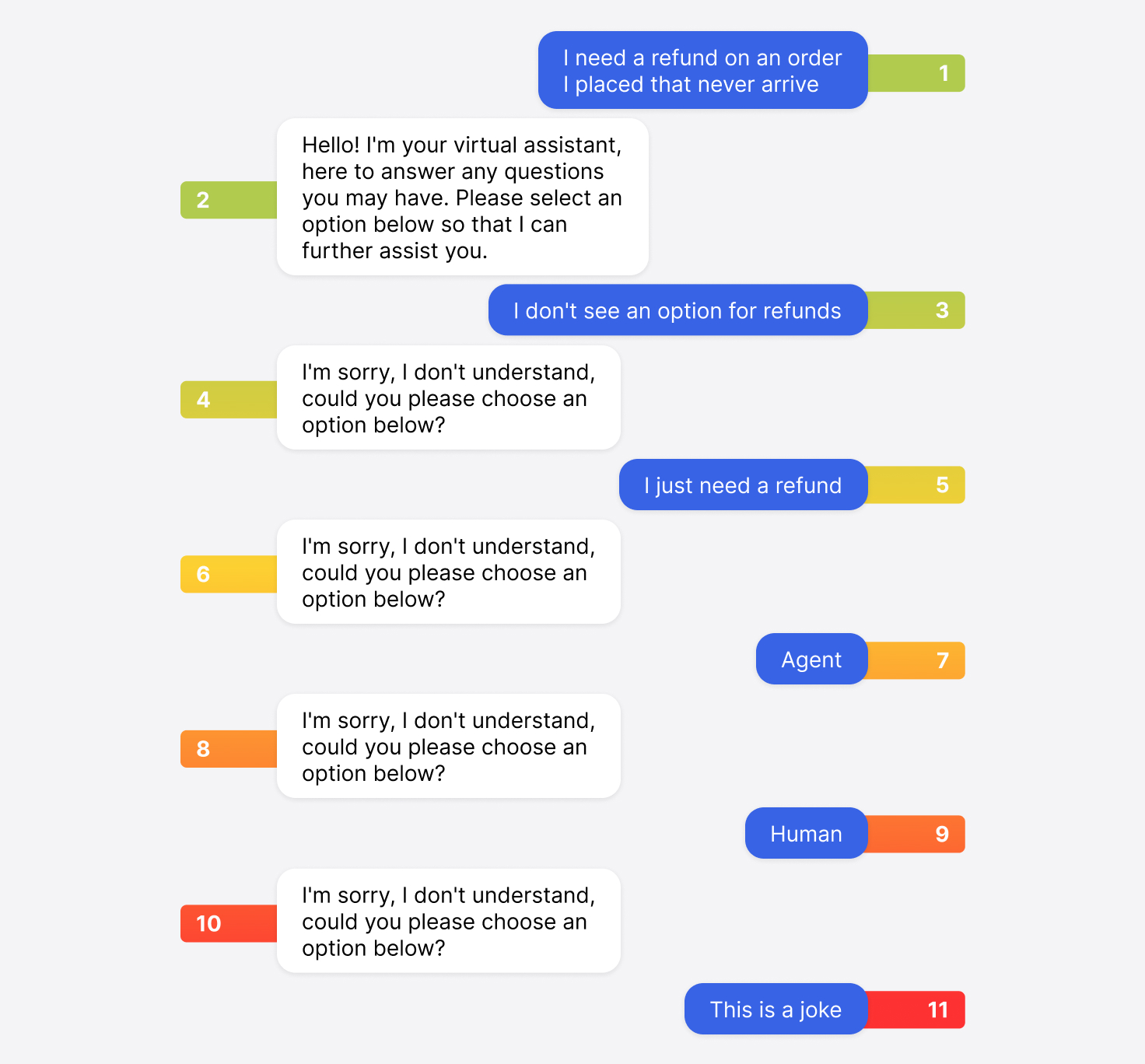
We see examples of bots failing like this too often. Bot responses follow specific scripts and can’t always react to consumers, resulting in negative interactions. You might think these problems are identifiable with traditional metrics, but that’s not the case.
The problems with using the following KPIs for chatbot analytics:
Containment conversation metrics
If the brand agent is a bot, and no human agent participates in the conversation, the user interaction is considered contained. The problem is this KPI doesn’t account for resolution. So, while the user engagement rate example above was fully contained by the bot, it obviously was not resolved.
Many automation programs are designed to prevent a consumer from reaching a live agent. In these cases, containment may be a good measurement for brand success, but does not truly reflect the consumer or customer experience itself.
Customer satisfaction surveys
Customer satisfaction (CSAT) and Net Promoter Score (NPS) are popular chatbot metrics for performance. However, collecting customer satisfaction scores can be a flawed process as it usually requires customers to complete surveys. In an interaction such as the example above, the customer never received the survey because the conversation was technically not completed. Additionally, Delighted tells us, “Depending on the channel used, the average customer survey response rate ranged from 6% to 16% in 2021.” With those rates, the data cannot be reliable or representative.
Also, if the conversation escalates from a bot to a human agent, the customer satisfaction score could be skewed by the human interaction. If survey responses are based on that, and not entirely on the chatbot users’ experience, the data again becomes unreliable.
Sentiment to measure customer satisfaction and emotion
LivePerson has a sentiment scoring algorithm called the Meaningful Conversation Score (MCS). The great thing about measuring sentiment is it applies to every message the customer sends, and is not dependent on surveys, bot vs. human agents, or agent behavior. This makes it an effective conversational chatbot analytics tool to help identify, based on language used, the consumer’s emotion throughout the conversation.
Unfortunately, sentiment cannot identify customer emotion accurately in automated bot responses.
In our example, the customer’s texts become short (often one word) and neutral in sentiment. Even the negative statement — “This is a joke” — can be interpreted either positively or negatively by the sentiment engine. Bot designs also commonly use guided flows in scripts, where the bot gives the customer a set of options. So, when the response is achieved with the click of a button, sentiment will always be neutral. Basically, people text differently to bots, with short, neutral sentiment responses, making sentiment analysis an incomplete metric for measuring bot performance and user satisfaction.
Transfer rate across the chatbot customer journey
This refers to how often a live agent gets involved, a common KPI used for chatbot analytics. But there are three challenges with this measurement:
- Abandoned conversations, like our example above, do not reflect in the transfer rate score because a live agent never got involved.
- Making a transfer is the goal for some bots.
- This measurement also doesn’t account for a customer asking for a human agent to start the conversation, which reflects the customer’s state of mind rather than the chatbot effectiveness, and inaccurately inflates negative measurement.
For example, a routing bot should have a 100% transfer rate, and a task-oriented bot (i.e., what is my order status) should have a significantly lower rate. Bot metrics like this require us to measure each chatbot separately for the most valuable insight, a cumbersome process when managing a large automation program.
Why use chatbot analytics?
Chatbot analytics are paramount in the world of digital engagement, offering insights essential for refining the user experience and guiding strategic business decisions. Businesses use chatbot analytics to:
1. Enhance user satisfaction
Chatbot analytics provide invaluable insights into user interactions, allowing businesses to understand user behavior, preferences and pain points. By analyzing this data, organizations can tailor chatbot responses to enhance overall user experience, leading to increased satisfaction and engagement.
2. Optimize chatbot performance
Analytics enable a comprehensive evaluation of chatbot effectiveness through metrics like message volume, engagement rate, and goal conversion rate. Continuous monitoring and analysis help identify areas for improvement, allowing businesses to optimize chatbot designs for better performance.
3. Measure return on investment (ROI)
Tracking development costs, subscription fees, and support expenses alongside chatbot performance metrics allows organizations to measure the ROI of their chatbot implementation. This financial analysis ensures that the resources invested in chatbot technology yield tangible benefits and cost savings.
4. Make data-driven decisions
Chatbot analytics empower businesses to make informed, data-driven decisions across various aspects of their operations. From refining marketing strategies to optimizing sales processes, organizations can align their initiatives with user preferences, ensuring strategic decisions are based on real-time data.
The future of chatbot analytics in measuring and delivering excellent customer support
This shift in how we measure conversational interactions is nothing new. In the early days of asynchronous messaging, we had to redefine how we measure contact center agents because traditional metrics like average handle time and concurrency became outdated. Now, as we’re locked into the world of AI and chatbot automation, and rely more on bots to support customer engagement programs, we need to again redefine how we measure success — because traditional metrics just don’t work anymore.